Driving Discovery Through Data
Enhancing patient outcomes with real-time analysis of vital signs
Hospitals collect large amounts of valuable data, but analysis is often confined to narrow fields of research. To disrupt the trend, Children's Hospital of Philadelphia (CHOP) is building a stream computing platform – enabling it to apply real-time predictive models to vital signs, providing a new form of clinical decision support for clinicians.
Business Challenge
CHOP recognized that its massive volumes of physiological clinical data presented a major opportunity to improve patient outcomes if it could extract insights from the data in near-real time.
Transformation
The hospital is creating a stream computing platform to analyze thousands of vital sign readings in near-real time, enabling it to use proven predictive models to support clinical decision-making.
Results
Delivers real-time health insight to drive faster, more effective intervention
Helps clinicians spot trends and risk indicators
Enables better-informed decision-making for improved care outcomes
Business Challenge History
Delivering critical care to vulnerable patients
Today’s hospitals are equipped to measure patients more accurately than ever, but the sheer speed, volume and variety of data generated by monitoring devices means the vast majority of this information is neither stored nor analyzed. CHOP recognized a valuable opportunity to disrupt this trend by harnessing data from medical sensors to improve patient care.
John Martin, MBA, Senior Director of the Enterprise Analytics and Reporting team at Children's Hospital of Philadelphia, explained: “Since the introduction of electronic patient monitoring, clinicians have been using real-time information on vital signs at the bedside to inform their decisions about patient treatment. Because data capture and modelling tools have historically been complex and costly, this information has gone largely untapped for analytics. As the data science discipline and Internet of Things (IoT) matured, we knew that we had an unprecedented opportunity to collect, analyze and utilize this data to improve care outcomes.”
Luis Ahumada, Ph.D., Principal Information Scientist in the Enterprise Analytics and Reporting team at Children's Hospital of Philadelphia, continued: “Our surgeons routinely carry out life-saving operations on infants, and the majority of patients must be intubated before they can be placed under general anesthetic. Because their airways are so small, a key challenge is determining if the tube has been optimally placed. This is important, because the patient’s ventilation may be impaired if the endotracheal tube is too high or too low.
“If a physical examination cannot confirm the placement of a tube, one of the most accurate ways to verify its position is through an x-ray procedure. However, these x-ray procedures may delay surgery and introduce additional radiation exposure.”
“With stream computing, we can acquire, analyze and act on our data to improve care outcomes – we’re excited for what the future holds.”
Transformation Story
Harnessing the power of stream computing
To help improve outcomes, CHOP is building an enterprise-class stream computing cluster, enabling it to ingest, process and analyze thousands of unique data points at high speed.
As a first step, the hospital created a data warehouse to enable it to use historical data to develop and refine its models, and gradually transition from retrospective to real-time analysis.
“We are developing streaming analytics algorithms that can serve as a continuous monitor to identify acute changes in ventilation, such as main-stem intubation,” explained Ahumada. “The goal of developing these algorithms is to use them in clinical decision-support applications to improve safety for our most vulnerable patients.”
He continued: “We collaborated with clinicians and researchers to use historical data to develop a model in MATLAB to detect whether a patient had been intubated optimally. The current model analyzes a number of data points, the most important of which is the amount of carbon dioxide expelled at the end of the respiration cycle. We discovered that subtle variations in end tidal carbon dioxide levels—when combined with factors such as blood pressure—are a strong predictor of whether or not a tube has been optimally placed.”
CHOP feeds sensor readings from its ventilation monitors to a central medical device integration (MDI) layer, which assigns metadata and forwards the information to the stream computing platform.
William Nieczpiel, Development Manager in the Enterprise Analytics and Reporting team at Children’s Hospital of Philadelphia explained: “The frequency of our readings can vary anywhere from five seconds for end tidal carbon dioxide levels to five minutes for blood pressure measurements, and each device uses different time standards and formats. To account for these differences, assigning a single, accurate master time code to each piece of data is essential. Similarly, we must link raw data from our sensors with the identity of the patient, which we can also achieve in the MDI layer by combining the device serial number with information from our Epic electronic medical records solution.”
By feeding sensor data from 550 beds and thousands of monitors into its stream computing environment, CHOP’s initial pilot model will ultimately be able to identify when a patient has been fitted with a tube—rather than another type of breathing apparatus such as a mask—the type of tube, and if the tube has been positioned optimally.
“Our initial results have achieved 81% accuracy with a specificity of 80% and a sensitivity of 84% to date, and we are confident that this model will become the first of many invaluable tools for decision support,” commented Ahumada. “We co-developed the model with our anesthesia group, and they are already championing internal evaluation of the model as a clinical decision-support tool. Once our stream computing environment is live, we can begin the all-important quality assurance phase of the project.”
He added: “During our first pilot, we will be comparing the model’s predictions with the results of x-rays and physical examinations for tubes that may not be optimally placed, and using the results to validate the accuracy of the model. Although positioning errors are rare, minimizing their occurrence is a powerful way to improve care outcomes and we are excited to move the model into production as an additional method of decision support for clinicians.”
Results Story
Improving care outcomes with analytics
With an enterprise stream computing environment delivering timely, accurate guidance to its clinicians, CHOP is confident that it can deliver even greater improvements to patient care within the tenets of clinical decision support.
“By comparing tiny variations in vital signs that would be extremely difficult for a human to detect, our pilot model is able to predict at a high level of specificity and sensitivity when tube placement is not optimal within the first five minutes of intubation,” said Ahumada.
Nieczpiel added: “After the solution goes into production, we plan to deliver real-time alerts directly to screens in the patient’s room via our Epic records system, and/or targeted alerts via mobile devices or other modalities. Although our clinicians will ultimately have the final decision about whether or not a tube is placed optimally, we see that our model will be another safeguard to keep our care at outstanding levels.”
In the long term, CHOP’s aim is to create a versatile, enterprise-class stream computing platform that can support a wide variety of use cases.
“We anticipate that our endotracheal tube positioning model will have a very positive impact on patient care, and it’s just the beginning,” explained Ahumada. “The lessons we are learning today will enable us to combine other published research with real-time data to create smarter analytical models. The potential use cases are infinite, but we are already considering ways to operationalize published models for patient deterioration, sepsis prevention, length of stay reduction and others.”
“Analysis of medical device data will become more widespread and a significant trend in healthcare in the coming years,” said Martin. “With stream computing, we can acquire, analyze and act on our data to improve care outcomes – we’re excited for what the future holds.”
He concluded: “We would like to give special thanks to Ali Jalali, PhD, and Jorge Galvez, MD, from Children’s Hospital of Philadelphia – Biomedical Informatics in Anesthesia and Critical Care department for their exceptional contributions to the project.”
About Children's Hospital of Philadelphia
Founded in 1855 in Pennsylvania, Children's Hospital of Philadelphia (CHOP) was the first hospital in the United States devoted exclusively to the care of children. One of the top-rated children's hospitals on the U.S. News & World Report's Honor Roll, the organization now employs more than 10,000 people and specializes in a range of pediatric disciplines, including oncology, cardiology and cardiothoracic surgery, airway disorders, and transplants.
Solution Components
- BigInsights
- HC: Collaborative Care and Health Information Exchange
- InfoSphere Streams
- PureData System for Analytics (Powered by Netezza Technology)
- PureData System for Analytics N1001-002
- Streams
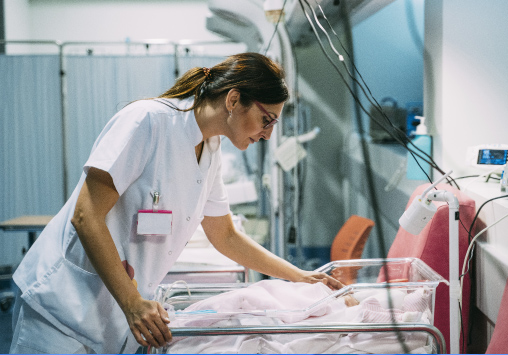
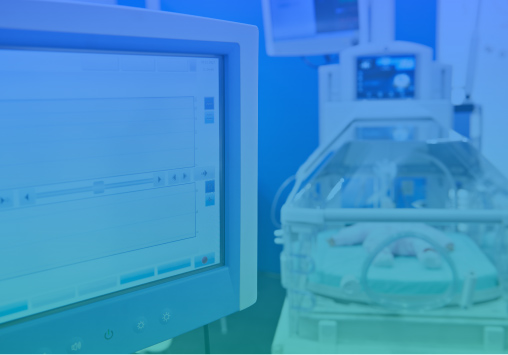